Research
Synthesizing Stealthy Attacks on Cardiac Devices
An Implantable Cardioverter Defibrillator (ICD) is a medical device for the detection of fatal cardiac arrhythmias and their treatment through electrical shocks intended to restore normal heart rhythm. An ICD reprogramming attack seeks to alter the device parameters to induce unnecessary therapy or prevent required therapy.
We present a formal approach for the synthesis of ICD reprogramming attacks that are both effective, i.e., lead to fundamental changes in the required therapy, and stealthy, i.e., are hard to detect. We focus on the discrimination algorithm underlying Boston Scientific devices (one of the principal ICD manufacturers) and formulate the synthesis problem as one of multi-objective optimization. Our solution technique is based on an Optimization Modulo Theories (OMT) encoding of the problem and allows us to derive device parameters that are optimal with respect to the effectiveness-stealthiness tradeoff. Our method can be tailored to the patients current condition, and generalizes to unseen signals.
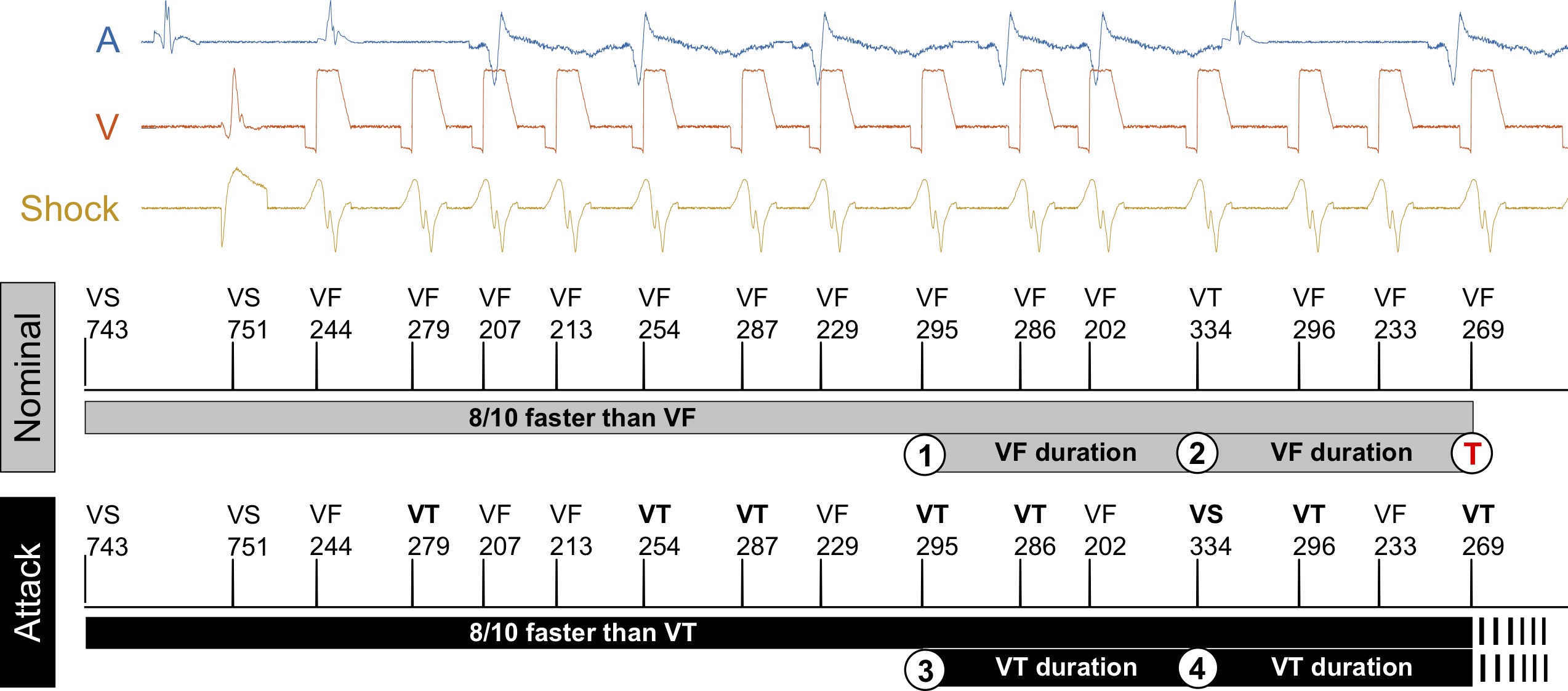
SMT-based synthesis of safe and robust digital controllers
We present a new method for the automated synthesis of digital controllers with formal safety guarantees for systems with nonlinear dynamics, noisy output measurements, and stochastic disturbances. Our method derives digital controllers such that the corresponding closed-loop system, modeled as a sampled-data stochastic control system, satisfies a safety specification with probability above a given threshold. The method alternates between two steps: generation of a candidate controller (sub-optimal but rapid to generate), and verification of the candidate. The candidate is found by maximizing a Monte Carlo estimate of the safety probability, and by simulating the system with a non-validated ODE solver. To rule out unstable candidate controllers, we prove and utilize Lyapunov indirect method for instability of sampled-data nonlinear systems. In the verification step, we use a validated solver based on SMT to compute a numerically and statistically valid confidence interval for the safety probability of the candidate controller. If such probability is not above the threshold, we expand the search space for candidates by increasing the controller degree. We evaluate our technique on three case studies: an artificial pancreas model, a powertrain control model, and a quadruple-tank process.
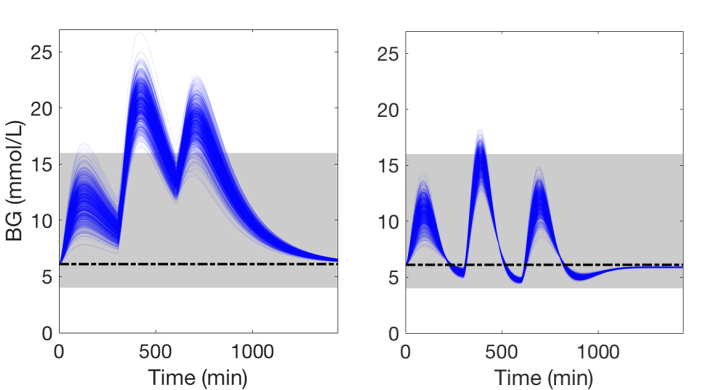
Optimal synthesis of stochastic chemical reaction networks
The automatic derivation of Chemical Reaction Networks (CRNs) with prescribed behavior is one of the holy grails of synthetic biology, allowing for design automation of molecular devices and in the construction of predictive biochemical models.
In this work, we provide the first method for optimal syntax-guided synthesis of stochastic CRNs that is able to derive not just rate parameters but also the structure of the network. Borrowing from the programming language community, we propose a sketching language for CRNs that allows to specify the network as a partial program, using holes and variables to capture unknown components. Under the Linear Noise Approximation of the chemical master equation, a CRN sketch has a semantics in terms of parametric Ordinary Differential Equations (ODEs).
We support rich correctness properties that describe the temporal profile of the network as constraints over mean and variance of chemical species, and their higher order derivatives. In this way, we can synthesize networks where e.g., one of the species exhibit a bell-shape profile or has variance greater than its expectation.
We synthesize CRNs that satisfies the sketch constraints and a correctness specification while minimizing a given cost function (capturing the structural complexity of the network). The optimal synthesis algorithm employs SMT solvers over reals and ODEs (iSAT) and a meta-sketching abstraction that speeds up the search through cost constraints.
We evaluate the approach on a three key problems: synthesis of networks with a bell-shaped profile (occurring in signaling cascades), a CRN implementation of a stochastic process with prescribed levels of noise and synthesis of sigmoidal profiles in the phosphorelay network.
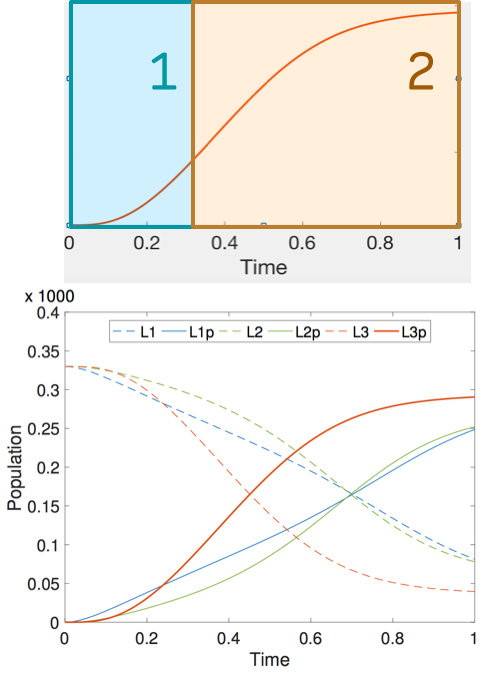
SMT-based synthesis of gene regulatory networks
Unraveling the structure and the logic of gene regulation is crucial to understand how organisms develop and so is the derivation of predictive models able to reproduce experimental observations and explain unknown biological interactions.
This research centers on the synthesis of biological programs, with specific focus on Boolean gene regulatory networks (GRN). We developed methods based on the idea of synthesis by sketching, which enables explicit modeling of hypotheses and unknown information, specified as e.g. choices or uninterpreted functions. Through the formalization as an SMT problem, the method can automatically and efficiently resolve the unknown information in order to obtain a model that is consistent with observations.
We applied this approach to synthesize the first GRN model of the sea urchin embryo (an important model organism in biology) that precisely and fully reproduce available temporal and spatial expression data and the effects of perturbation experiments. The data we used is the result of 30+ years of research in the Davidson lab at Caltech.
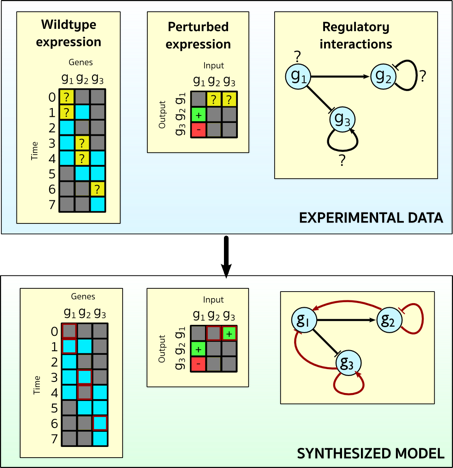
Parameter synthesis for pacemaker design optimization
Verification is useful in establishing key correctness properties of cardiac pacemakers, but has limitations, in that it is not clear how to redesign the model if it fails to satisfy a given property. Instead, parameter synthesis aims to automatically find optimal values of parameters to guarantee that a given property is satisfied.
In this project, we develop methods to synthesize pacemaker parameters that are safe, robust and, at the same time, able to optimise a given quantitative requirement such as energy consumption or clinical indicators. We solve this problem by combining symbolic methods (SMT solving) for ruling out parameters that violate heart safety (red areas in the figure) with evolutionary strategies for optimising the quantitative requirement.
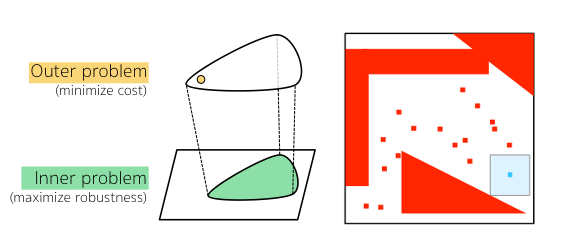