Research
Machine learning for Predictive Monitoring of Cyber-Physical Systems
Formal verification of Cyber-Physical Systems (CPSs) typically amount to model checking of some formal model (a hybrid automaton) of the CPS. However, this task is computationally expensive, and so is limited to offline analysis. Moreover, the strong guarantees of model checking often cease to hold at runtime due to uncertain operating environments and complex processes that are hard to model precisely.
We focus on the predictive monitoring of CPSs, i.e., the problem of predicting at runtime if a safety violation can be reached from the current CPS state. In order to provide scalable and accurate predictive monitors, we propose Neural Predictive Monitoring (NPM) a machine learning-based approach based on learning reachability predictors described by deep neural networks, aka neural state classifiers, using supervision from a hybrid automata reachability checker. To prevent potentially harmful prediction error, NPM includes methods to quantify the predictive uncertainty of the monitor, and uses these uncertainty measures to derive rejection criteria that optimally recognize such errors, without knowing the true reachability value. Finally, NPM includes an active learning method that uses such uncertainty measures to re-train and improve the monitor and the rejection rule where it most matters, i.e., on the uncertain states. NPM empirically shows great performance: it is efficient, with computation times on the order of milliseconds, and effective, with highly accurate monitors and high error recognition rates.

Probabilistic Robustness of Bayesian Neural Networks
We introduce a probabilistic robustness measure for Bayesian Neural Networks (BNNs), defined as the probability that, given a test point, there exists a point within a bounded set such that the BNN prediction differs between the two. Such a measure can be used, for instance, to quantify the probability of the existence of adversarial examples. Building on statistical verification techniques for probabilistic models, we develop a framework that allows us to estimate probabilistic robustness for a BNN with statistical guarantees, i.e., with a priori error and confidence bounds, by leveraging a sequential probability estimation scheme based on the Massart bounds.
We evaluate our method with several approximate BNN inference techniques on image classification tasks associated to the MNIST digit recognition dataset and a two-class subset of the German Traffic Sign Recognition Benchmark (GTSRB) dataset, showing that the method enables quantification of uncertainty of BNN predictions in adversarial settings. Code available in GitHub.
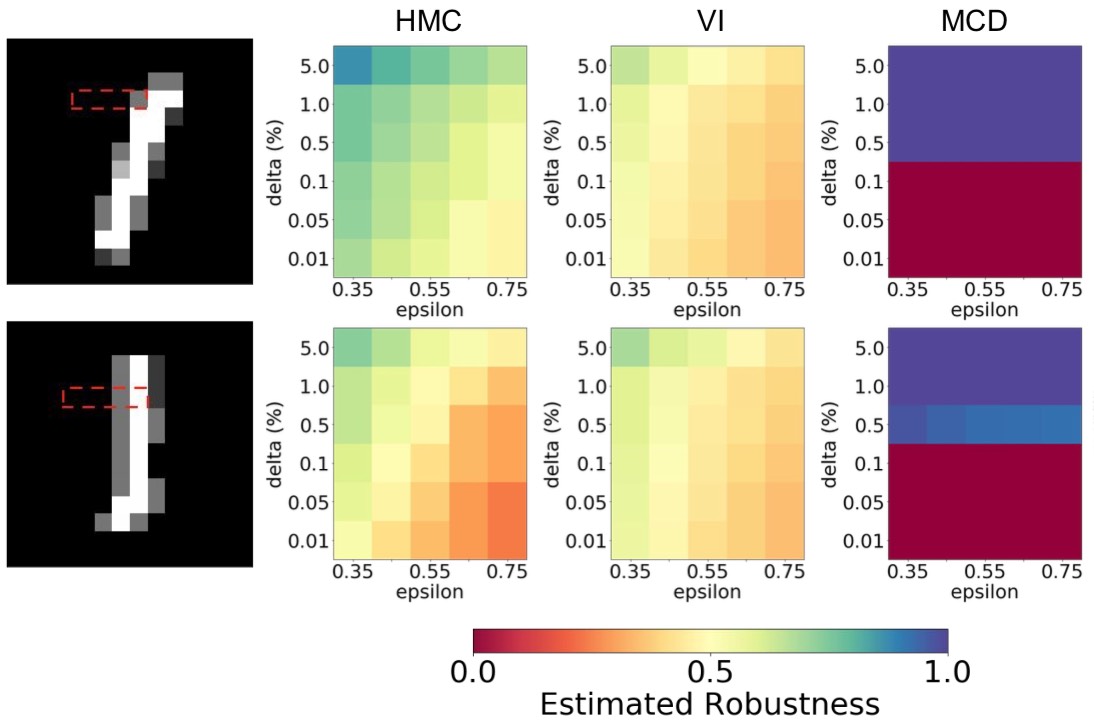
Closed-loop quantitative verification of rate-adaptive pacemakers
Rate-adaptive (RA) pacemakers are able to adjust the pacing rate depending on the levels of activity of the patient, detected by processing physiological signals data. RA pacemakers represent the only choice when the heart rate cannot naturally adapt to increasing demand (e.g. AV block). RA parameters depend on many patient-specific factors, and effective personalisation of such treatments can only be achieved through extensive exercise testing, which is normally intolerable for a cardiac patient.
We introduce a data-driven and model-based approach for the quantitative verification of RA pacemakers and formal analysis of personalised treatments. We developed a novel dual-sensor pacemaker model where the adaptive rate is computed by blending information from an accelerometer, and a metabolic sensor based on the QT interval (a feature of the ECG). The approach builds on the HeartVerify tool to provide statistical model checking of the probabilistic heart-pacemaker models, and supports model personalization from ECG data (see heart model page). Closed-loop analysis is achieved through the online generation of synthetic, model-based QT intervals and acceleration signals.
We further extend the model personalization method to estimate parameters from patient population, thus enabling safety verification of the device. We evaluate the approach on three subjects and a pool of virtual patients, providing rigorous, quantitative insights into the closed-loop behaviour of the device under different exercise levels and heart conditions.
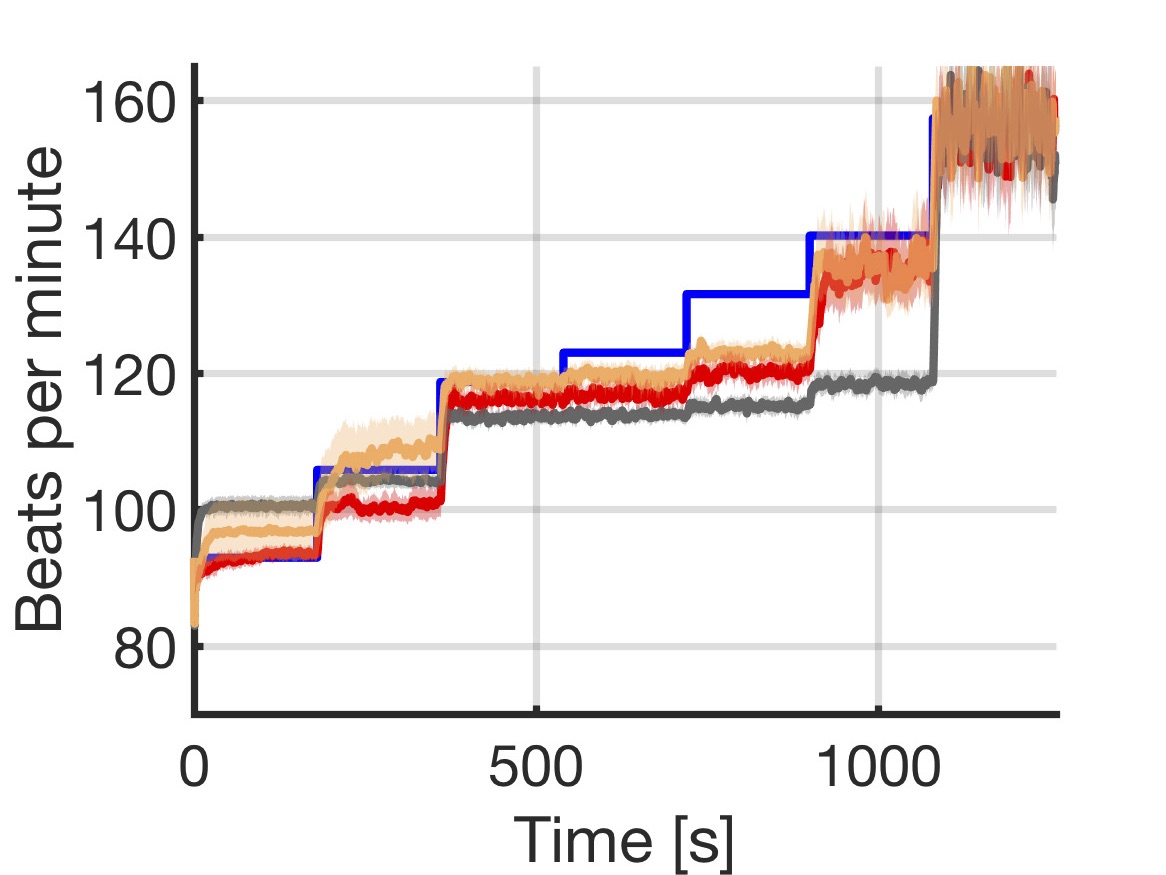
HeartVerify: Model-Based Quantitative Verification of Cardiac Pacemakers
HeartVerify is a framework for the analysis and verification of pacemaker software and personalised heart models. Models are specified in MATLAB Stateflow and are analysed using the Cosmos tool for statistical model checking, thus enabling the analysis of complex nonlinear dynamics of the heart, where precise (numerical) verification methods typically fail.
The approach is modular in that it allows configuring and testing different heart and pacemaker models in a plug-and-play fashion, without changing their communication interface or the verification engine. It supports the verification of probabilistic properties, together with additional analyses such as simulation, generation of probability density plots (see figure) and parametric analyses. HeartVerify comes with different heart and pacemaker models, including methods for model personalization from ECG data and rate-adaptive pacing.
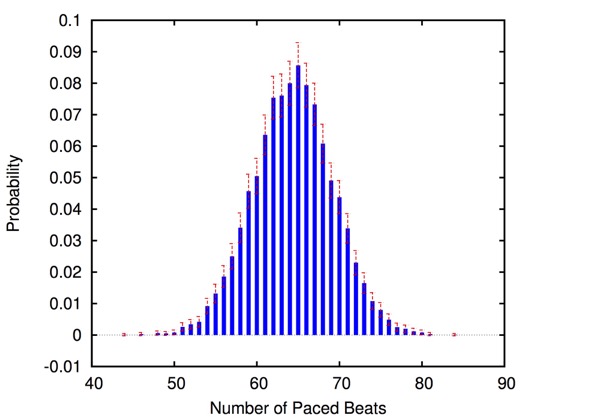
Probabilistic timed modelling of cardiac dynamics and personalization from ECG
We developed a timed automata translation of the IDHP (Integrated Dual-chamber Heart and Pacer) model by Lian et al. Timed components realize the conduction delays between functional components of the heart and action potential propagation between nodes is implemented through synchronisation between the involved components. In this way, the model can be easily extended with other accessory conduction pathways in order to reproduce specific heart conditions.
The IDHP model can be also parametrised from patient electrocardiogram (ECG) in order to reproduce the specific physiological characteristics of the individual. For this purpose, we extended the model in order to generate synthetic ECG signals that reflect the heart events occurring at simulation time. Starting from raw ECG recordings, our method automatically finds model parameters such that the synthetic signal best mimics the input ECG, by minimising the statistical distance between the two. The resulting parameters correspond to probabilistic delays in order to reflect variability of ECG features.
The IDHP heart model can be downloaded from the tool page, while personalization from ECG data is implemented in the HeartVerify tool.
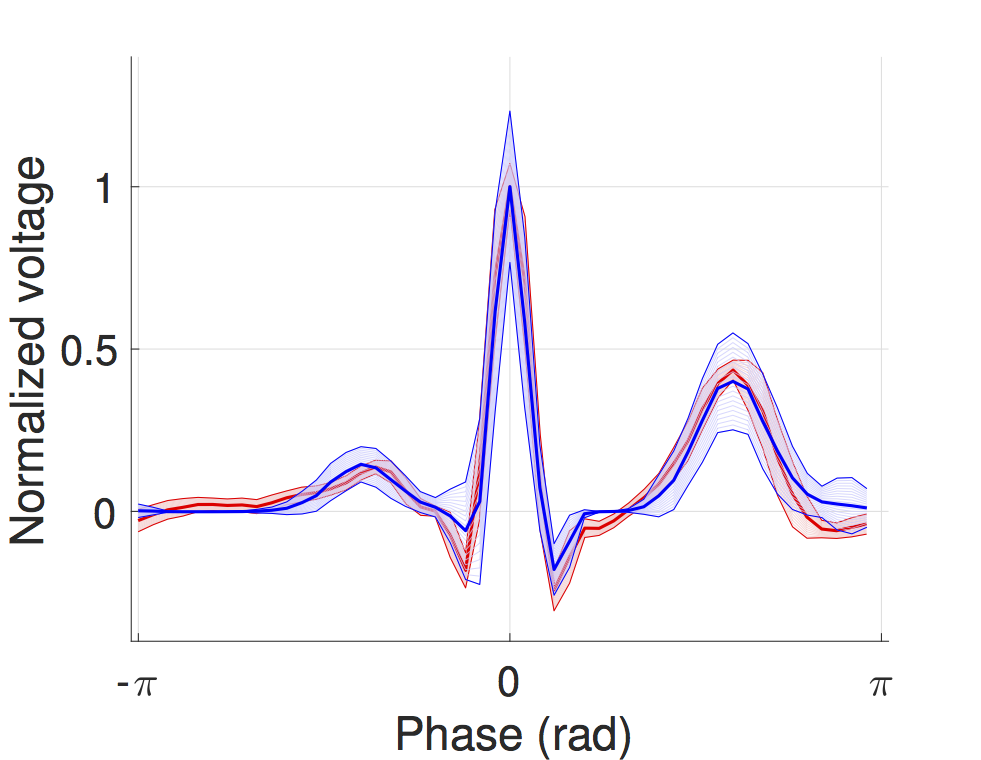
Formal analysis of bone remodelling
In this project we study bone remodelling, i.e., the biological process of bone renewal, through the use of computational methods and formal analysis techniques. Bone remodelling is a paradigm for many homeostatic processes in the human body and consists of two highly coordinated phases: resorption of old bone by cells called osteoclasts, and formation of new bone by osteoblasts. Specifically, we aim to understand how diseases caused by the weakening of the bone tissue arise as the resulting process of multiscale effects linking the molecular signalling level (RANK/RANKL/OPG pathway) and the cellular level.
To address crucial spatial aspects such as cell localisation and molecular gradients, we developed a modelling framework based on spatial process algebras and a stochastic agent-based semantics, realised in the Repast Simphony tool. This resulted in the first agent-based model and tool for bone remodelling, see also the Tools page.
In addition, we explored probabilistic model checking methods to precisely assess the probability of a given bone disease to occur, and also hybrid approximations to tame the non-linear dynamics of the system.
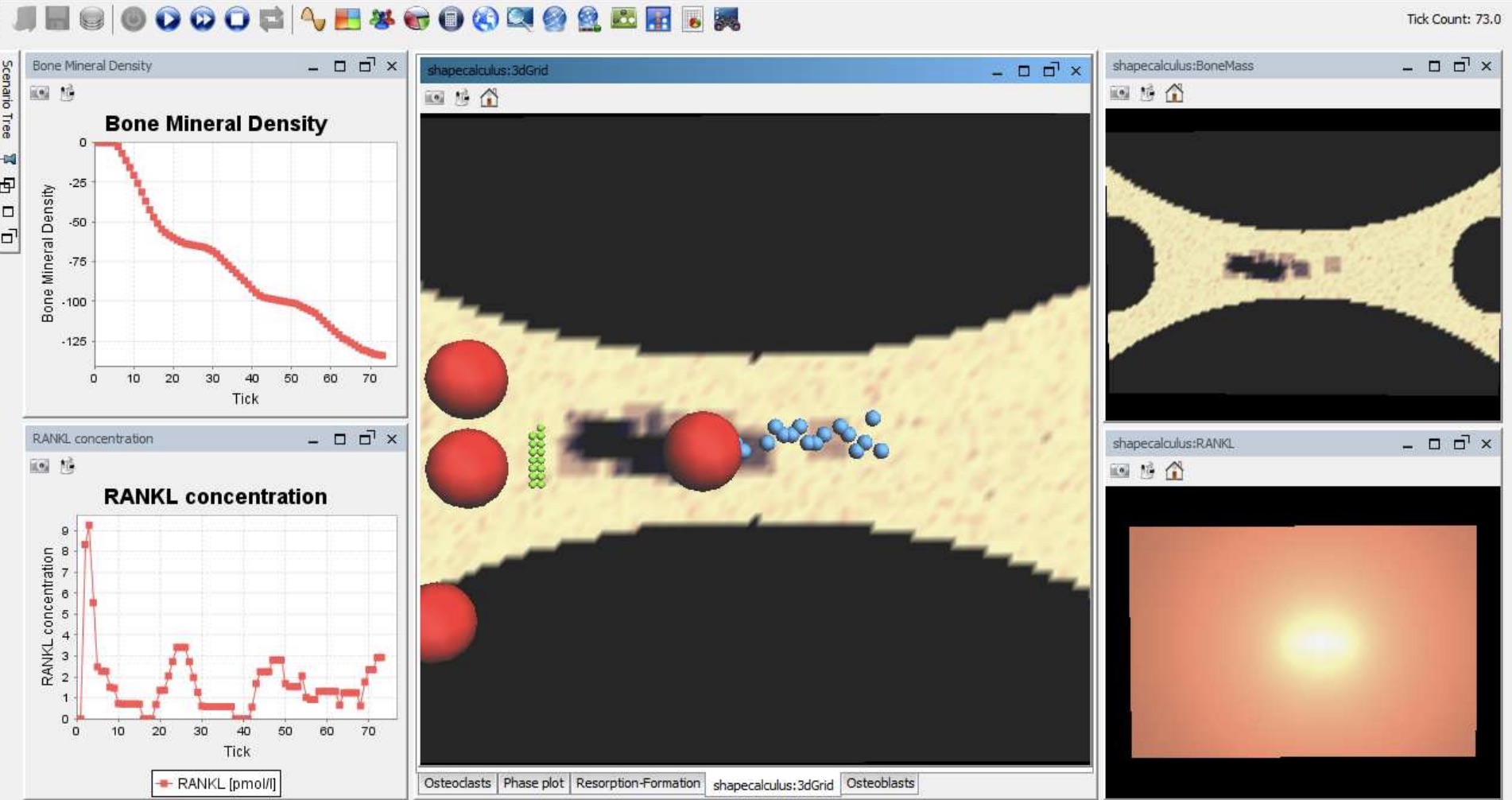
A multi-level model for self-adaptive systems
Self-adaptive systems are able to modify their own behaviour according to their environment and their current configuration, in order to fulfil an objective, to better respond to problems, or maintain desired conditions.
In this project, we introduce a hierarchical approach to formal modelling and analysis of self-adaptive systems. It is based on a structural level S, describing the adaptation dynamics of the system, and a behavioural level B describing the admissible dynamics of the system. The S level imposes structural constraints on the B level, which has to adapt whenever it no longer can satisfy them (top-down adaptation).
We introduce weak and strong adaptability relations, capturing the ability of a system to adapt for some evolution paths or for all possible evolutions, respectively, and show that adaptability checking, i.e. deciding if a system is weak or strong adaptable, can be reduced to a CTL model checking problem.
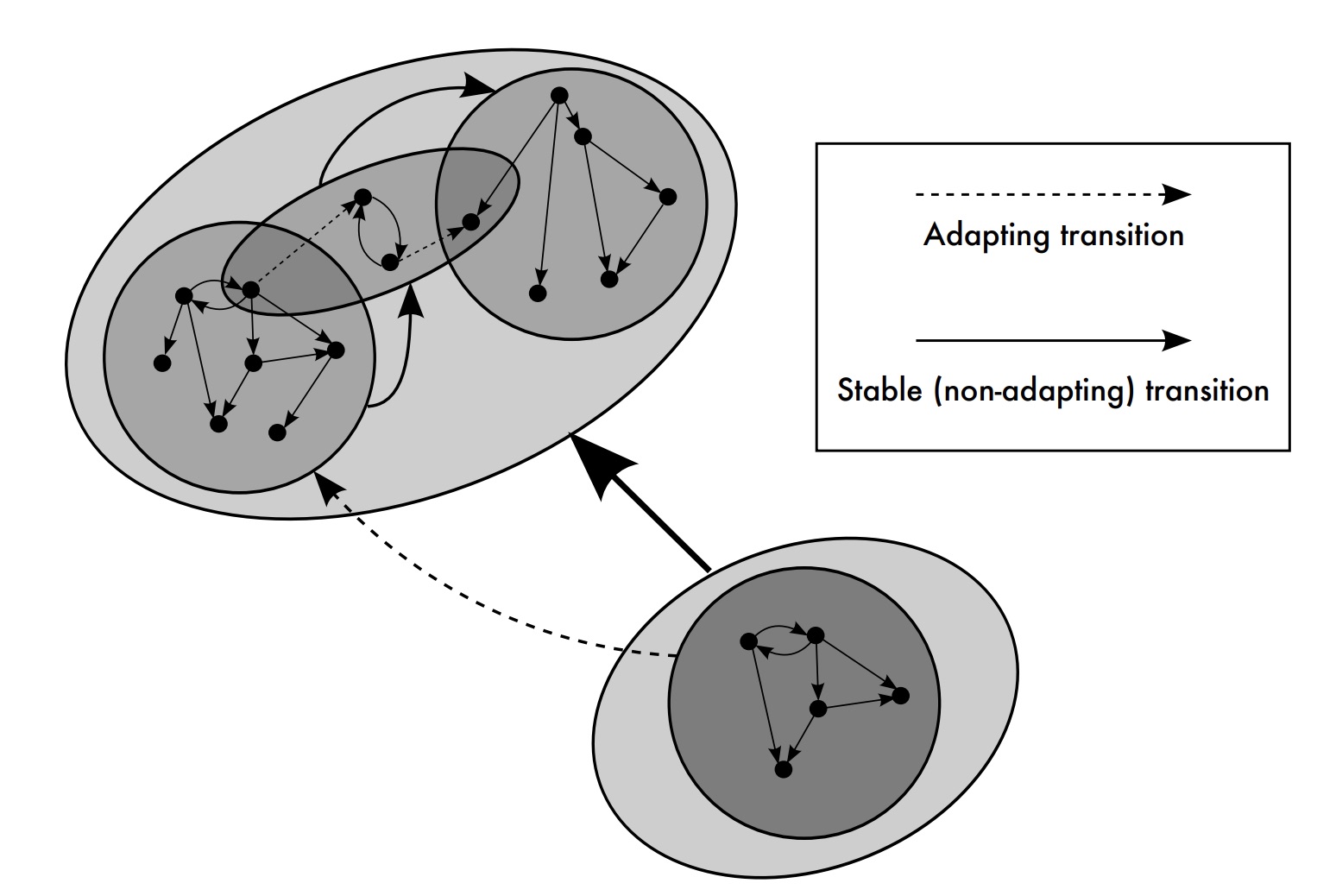